Data Lake
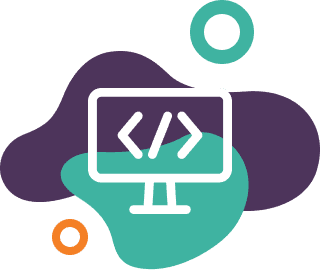
What is a Data Lake?
A Data Lake is a storage repository designed to hold vast amounts of raw data in its original form. Unlike traditional data warehouses that organize data into predefined structures, data lakes are more flexible, storing structured, semi-structured, and unstructured data without needing a predetermined format.
This flexibility makes data lakes particularly valuable for big data analytics, machine learning, and real-time data processing, where diverse types of data, such as sensor readings, social media feeds, or transaction records, can be analyzed together to provide comprehensive insights.
Structure and Key Characteristics
The architecture of a data lake typically follows a "schema-on-read" model. This means that data is stored as-is and can be structured only when it’s accessed for analysis, allowing users to explore data freely. This approach contrasts with the "schema-on-write" method used in data warehouses, which requires data to be formatted and structured before storage.
Common platforms that support data lake architectures include Amazon S3, Microsoft Azure, and Hadoop, offering scalable, cost-effective solutions that can handle petabytes of data.
Benefits of a Data Lake
One of the primary advantages of a data lake is its ability to handle various types of data formats simultaneously, including JSON, XML, images, and videos. This flexibility allows organizations to store and analyze a wide array of data sources in one place.
Data lakes also support real-time analytics, meaning users can work with up-to-the-minute data to make fast, informed decisions. Additionally, data lakes can scale on-demand, making them ideal for handling fluctuating data volumes and ensuring that storage needs are met cost-effectively.
Challenges of Using Data Lakes
- Despite their advantages, data lakes come with several challenges. One of the most common issues is the risk of creating a "data swamp." Without careful governance, data lakes can accumulate redundant, unused, or unorganized data, which can diminish the lake's efficiency and usability.
- Data quality and consistency are other concerns, as the more sources added to the lake, the harder it is to maintain data integrity. Implementing robust access controls, data cataloging, and metadata management solutions are essential to keeping the data lake functional and valuable.
The Future of Data Lakes
Data lakes continue to evolve, driven by advancements in artificial intelligence and machine learning. The next generation of data lakes integrates seamlessly with data warehouses, allowing organizations to balance the flexibility of a data lake with the structured storage of a warehouse.
Improved governance solutions are also emerging to help prevent data swamps, offering organizations better ways to manage and retrieve data. This development ensures that data lakes remain a powerful tool for organizations aiming to harness big data for competitive advantage in fields like AI, predictive analytics, and IoT.